Modelling Customer Behaviour in Supermarkets
- Researcher: Fabian Ying
- Academic Supervisors: Sam Howison, Mariano Beguerisse and Mason Porter (UCLA)
- Industrial Supervisors: Alisdair Wallis, Trevor Sidery and Robert Armstrong
Background
The aim of this project is to develop a model that can be used to describe, predict and reduce congestion in UK supermarkets.
The grocery retail market is a highly competitive sectorand thus anything that improves the store for customers is of great interest to retailers such as Tesco. Identifying and reducing congestion will both enhance the shopping experience for customers and reduce the cost to fulfill online or-ders from within the store.
We use anonymised ordered customer-basket data inside supermarkets to develop and calibrate our models
Outcomes
To predict congestion under new store layouts, we first need a model thatcan predict how customers move in new store layouts. To do this, we use human mobility models, which have been used to describe and predict traffic flow in cities, commuting flow between states/counties, and trade flows between countries. With these models, we predict the mobility flow of customers between any two zones in the store based on the popularity of items in the zones and the distance between the zones.
We infer the empirical mobility flow from anonymized ordered customer-basket data, and we find that several models (including the gravity model) can successfully predict on average 65% to 70% of the empirical mobility flow across 17 stores. From the mobility flow, we can then estimate the number of visits (assuming shortest walk routing between purchases) that a node receives and calculate measures of congestion based on this. We also find that the models give excellent agreement with the number of visits that are estimated from the empirical flow (see Figure 1).
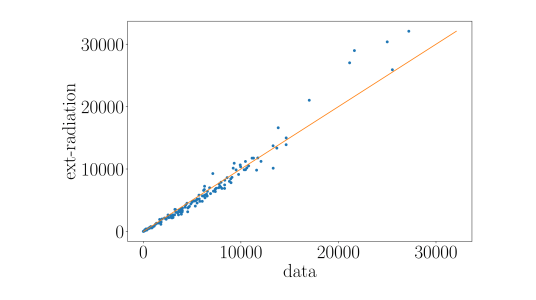
Figure 1: Comparison between the number of visits to each node calculated from our model and from the data. The orange line is the identity.
Finally, we use a simple optimization algorithm (called simulated annealing) to find store layouts with less congestion. We assume that the basic geometry of the store is fixed and that we can only swap aisles of the size length with one another. We find that our optimized store layouts have up to 50% lower congestion values and disperse popular across the store (see Figure 2).
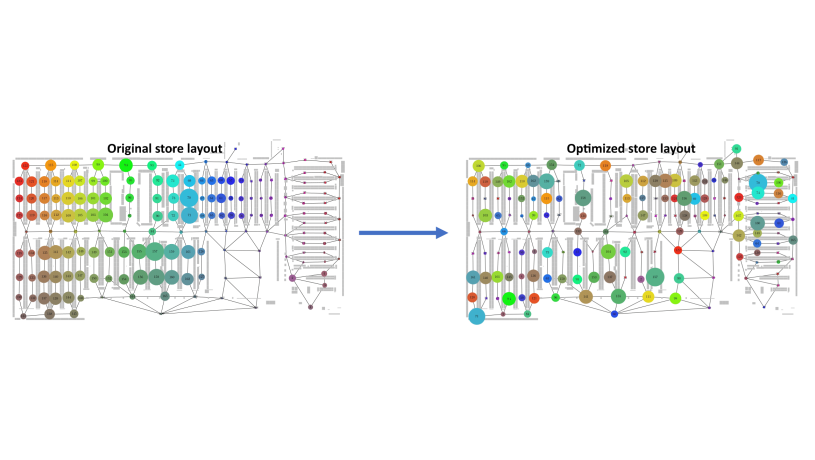
Figure 2: Comparison between original store layout and optimized store layout. Nodes of the same colour (except grey) belong to the same aisle. Grey nodes do not belong to any aisle.The node size is proportional to the popularity of the node (measured by the number of purchases made inthere).The entrance and till nodes are circled in yellow and red, respectively
Hence our work has shown promising results for using human mobility models to predict congestion and using simple optimization algorithms to find store layouts with less congestion. Our modelling framework can also be easily extended to incorporate more realistic routing models for customers or more realistic congestion models. Furthermore, our work is the first (to the best of our knowledge) that has successfully applied these mobility models to small spatial scales.
Publications
F. Ying, A. O. G. Wallis, M.Beguerisse-Díaz, M. A. Porter, S. D. Howison. “Customer mobility and congestion in supermarkets.” (2019), arXiv:1905.13098. (under review)