One of the greatest challenges in cancer treatment is maximising the response to a given drug - how can doctors get the greatest impact for the patient from the drug?
Traditionally, the answer to this has been ‘maximum tolerated dose’ (MTD) therapy, where the patient continually receives a high drug dose, with no breaks in treatment.
However, researchers and clinicians at the Moffitt Cancer Centre (Tampa, Florida) have proposed an alternative approach for late-stage cancers that are partially resistant to treatment [1]. By introducing breaks in the treatment (Figure 1), they allowed cells that are sensitive to drug treatment to regrow, so that the tumour as a whole would continue to respond to the drug and remain under control for longer.
Figure 1:Illustration of the designed evolutionary dynamics in adaptive therapy, reproduced from [1]. The purple cells are sensitive to the treatment and the green cells are resistant, with their respective densities plotted over time during treatment. Maximum tolerated dose therapy is given in (a), quickly eliminating cells resistive to the treatment mechanism, which allows resistant cells to grow unchecked. In (b), therapy is halted before all of the sensitive cells are eliminated, so that they will continue to compete with the resistant cells, delaying patient relapse.
In a new study published in Cancer Research, Oxford Mathematicians Kit Gallagher and Philip Maini, alongside collaborators at the Moffitt Cancer Center, introduce a novel framework that leverages deep reinforcement learning (DRL) to personalise the timing of these breaks in treatment for individual prostate cancer patients, potentially doubling the time to relapse compared to MTD or non-personalised treatment breaks.
They trained the deep reinforcement learning network on synthetic data from a mathematical model of prostate cancer, created by a prior Oxford Mathematician Maximilian Strobl [2] to replicate behaviour seen in previous clinical trials [3]. The mathematical model was vital to generate sufficiently large quantities of ‘virtual patient’ data and allowed the researchers to evaluate treatment schedules that couldn’t easily be tested clinically.
To translate this work into clinical practice, they extracted interpretable treatment strategies from the ‘black-box’ deep learning network, which a clinician would be able to prescribe to their patients. So that this approach could also support patients starting new drugs (where a doctor has no history of their response to that drug and so can’t recommend a personalised schedule), the researchers proposed a five-step pathway (Figure 2), wherein patients would initially undergo a standardised treatment cycle. A ‘virtual twin’ of this patient would then be created based on their data from this initial treatment, which could be used to finetune the DRL model so that it could generate a personalised treatment schedule. These schedules consistently outperform clinical standard-of-care protocols as well as generic adaptive therapy, demonstrating how the results from this computational study could be translated to support clinical decision-making.
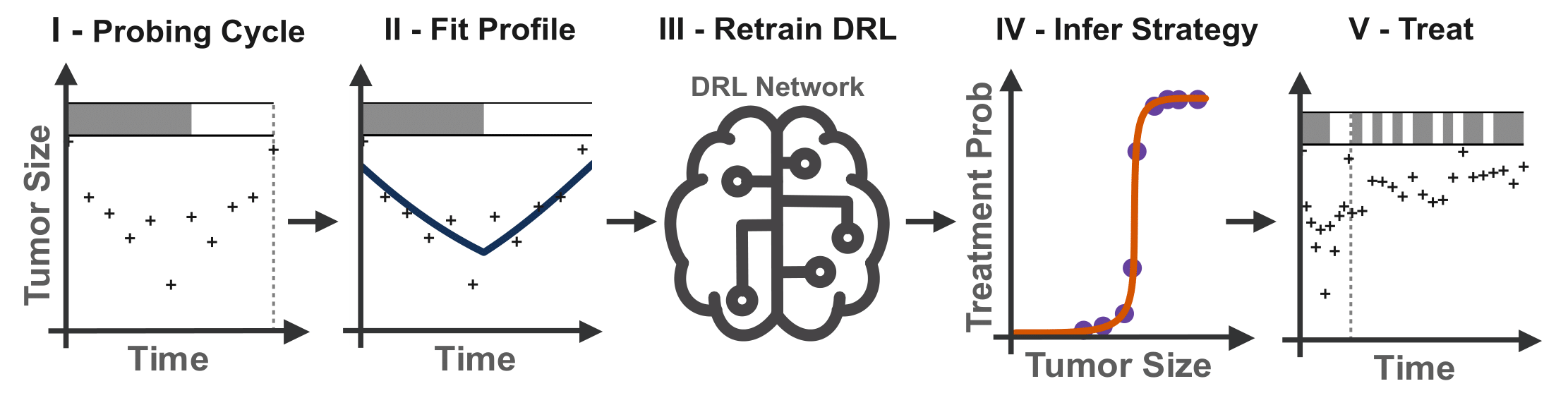
Figure 2: Patients undergo an initial “probing” cycle of conventional adaptive therapy, to which the virtual patient model is fitted, generating a set of tumour parameters specific to each patient. A copy of the generalised DRL model is then retrained on these personalised parameters, fine-tuning the DRL network to that patient’s treatment response. Finally, a rational treatment strategy is extracted from the individual’s DRL model, providing personalised recommendations throughout the remainder of the treatment schedule.
You can watch Kit talking about his work here.
References:
[1] Zhang J, Cunningham JJ, Brown JS, Gatenby RA. Integrating evolutionary dynamics into treatment of metastatic castrate-resistant prostate cancer. Nature communications. 2017 Nov 28;8(1):1816.
[2] Strobl MA, West J, Viossat Y, Damaghi M, Robertson-Tessi M, Brown JS, Gatenby RA, Maini PK, Anderson AR. Turnover modulates the need for a cost of resistance in adaptive therapy. Cancer research. 2021 Feb 15;81(4):1135-47.
[3] Bruchovsky N, Klotz L, Crook J, Malone S, Ludgate C, Morris WJ, Gleave ME, Goldenberg SL. Final results of the Canadian prospective phase II trial of intermittent androgen suppression for men in biochemical recurrence after radiotherapy for locally advanced prostate cancer: clinical parameters. Cancer. 2006 Jul 15;107(2):389-95.