15:00
Approximate lattices: structure and beyond
Abstract
Approximate lattices are aperiodic generalisations of lattices in locally compact groups. They were first introduced in abelian groups by Yves Meyer before being studied as mathematical models for quasi-crystals. Since then their structure has been thoroughly investigated in both abelian and non-abelian settings.
In this talk I will survey what is known of the structure of approximate lattices. I will highlight some objects - such as a notion of cohomology sitting between group cohomology and bounded cohomology - that appear in their study. I will also formulate open problems and conjectures related to approximate lattices.
17:00
Mobilizing Mathematics for the Fight Against Cancer - Trachette Jackson
Mathematical oncologists apply mathematical and computational models to every aspect of cancer biology, from tumor initiation to malignant spread and treatment response. A substantial amount of medical research now focuses on the molecular biology of individual tumors to selectively target pathways involved in tumor progression, leading to careful manipulation of these pathways, and new cell-specific approaches to cancer therapy are now being developed. At the same time, advances in cancer immunotherapies have led to a reemergence of their use and effectiveness. Using data-driven computational models is a powerful and practical way to investigate the therapeutic potential of novel combinations of these two very different strategies for clinical cancer treatment.
Trachette will showcase mathematical models designed to optimize targeted drug treatment strategies in combination with immunotherapy, to gain a more robust understanding of how specific tumor mutations affect the immune system and ultimately impact combination therapy. Combined with existing and newly generated experimental data, these models are poised to improve the ability to connect promising drugs for clinical trials and reduce the time and costs of transitioning novel therapeutic approaches from “equations to bench to bedside.”
Trachette Jackson is Professor of Mathematics at the University of Michigan and recipient of many awards for her work in her field and for her commitment to increasing opportunities for girls, women, and underrepresented minority students.
Please email @email to register to attend in person.
The lecture will be broadcast on the Oxford Mathematics YouTube Channel on Thursday 21 March at 5-6pm and any time after (no need to register for the online version).
The Oxford Mathematics Public Lectures are generously supported by XTX Markets.
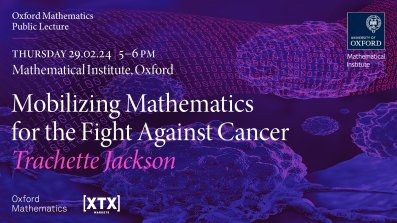