Speakers & Short Talks
Invited Speakers (A-Z)
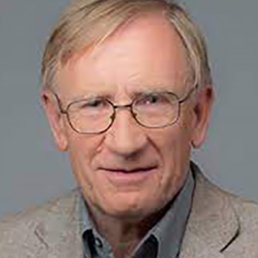
Gunnar Carlsson
Stanford University
The TDA User Experience
We will discuss methods for making TDA more easily usable, and methods we could develop which will turn it into a systematic tool for data analysis. Our methods are inspired both by the experience in "pure" topology as well as interaction with various data sets. We will include numerous examples.
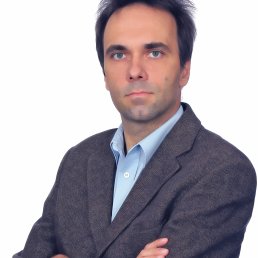
Paweł Dłotko
Dioscuri Centre in Topological Data Analysis, Polish Academy of Sciences
A Topological Toolbox in Data Science
Data and their analysis methods have an enormous impact on our everyday lives. In this talk we will present a selection of tools developed by my group at the Dioscuri Centre in Topological Data Analysis. We will start by exploring various ways to encapsulate the robust features of the shape of data including tools of Euler curves and profiles and their application in Goodness-of-Fit tests in statistics. We will subsequntly discuss how those and the standard features can be compared in an efficient and robust way. The talk will be concluded with topologically inspired methods for visualizing high-dimensional and complex data, helping us build intuition about the datasets at hand.
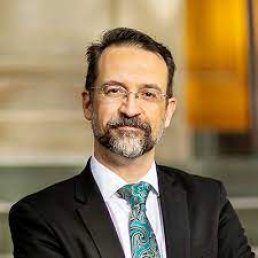
Rob Ghrist
University of Pennsylvania
Harmonic Methods for Sheaves and Data
There are numerous challenges in topological data analysis which concern the passage from local data, relationships, or constraints, to global information, systems, or solutions. This talk will highlight a branch of algebraic topology -- sheaf theory -- uniquely suited to address such challenges. Particular emphasis will be placed on novel methods in cellular sheaf theory which can be called "harmonic". Based on Hodge theory and sheaf Laplacians, these methods are local, computationally tractable, and effective in characterizing global features. Also addressed is the generalization of these methods from vector-valued data with linear constraints to lattice-valued data with nonlinear-but-ordered structure.
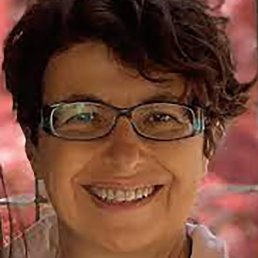
Claudia Landi
Università degli Studi di Modena e Reggio
Tame functors from posets to chain complexes
Tame functors indexed by posets with values in the category of non-negative chain complexes are a case of generalized persistence modules convenient for encoding homotopical and homological properties of objects in topological data analysis. We will start discussing some circumstances in which the category of such functors admits both an Abelian and a model structure, highlighting the case of posets of dimension 1. Next, we will consider structure theorems in this category. We will see that the structure theorem for filtered (i.e. with internal maps that are monomorphisms) tame functors indexed by non-negative reals gives insights into the usual persistent homology barcoding algorithm. In the case of factored (i.e. with internal maps that are epimorphisms) tame functors, again indexed by non-negative reals, the structure theorem yields the construction of barcodes for Morse-Smale vector fields. Generally, when functors need not be filtered nor factored, the family of indecomposables is wild. However, if the indexing poset is of dimension 1, functors admit a cofibrant replacement for which a structure theorem will be presented.

Anthea Monod
Imperial College, London
Reflections on Persistent Homology in Statistics, Machine Learning, and AI
A central tool in topological data analysis, persistent homology has made appearances over the years in wide-reaching applications, positioning itself in many instances as a cutting-edge data-scientific methodology. In this talk, I will attempt to overview and reflect on the role and development of persistent homology in practice over the years, especially in the parallel development and advancement of data analytic fields from statistics to machine learning to the current landscape of deep learning and artificial intelligence.
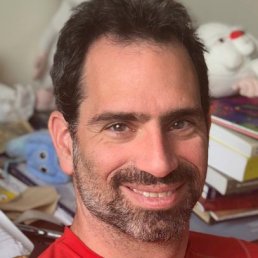
Ezra Miller
Duke University
New horizons in algebra for multiparameter persistence
Persistent homology modules in one parameter decompose uniquely up to isomorphism as direct sums of interval modules, which are indecomposable. Ideally, this kind of positive comparison between arbitrary multipersistence modules and interval modules would extend to multiple parameters, but arbitrary modules do not decompose as direct sums of interval modules; only a small subset of the indecomposables are interval modules. Much research over the past decade on multiparameter persistence has therefore focused on (a) retaining positivity by unique direct sum decomposition into indecomposables, sacrificing the connection to interval modules, or (b) retaining the connection to interval modules, which are easy to describe and interpretable, using signed homological or virtual methods. With applications in mind, this research has paid close attention to stability under perturbation with respect to natural distances between modules, most notably interleaving distance. Can the apparently incompatible ideals of positivity and comparison with interval objects be achieved simultaneously? Perhaps. Familiar objects from algebra, geometry, and topology such as groups, coherent sheaves, or vector bundles, can't be expected to -- and don't, generally speaking -- break into direct sums of simple objects, either in the mathematical or ordinary English meaning of "simple". But arbitrary objects in these contexts can instead be filtered by simpler objects. This talk will review some of the history leading to this point and the promise that filtration might hold for the future of multiparameter persistence.
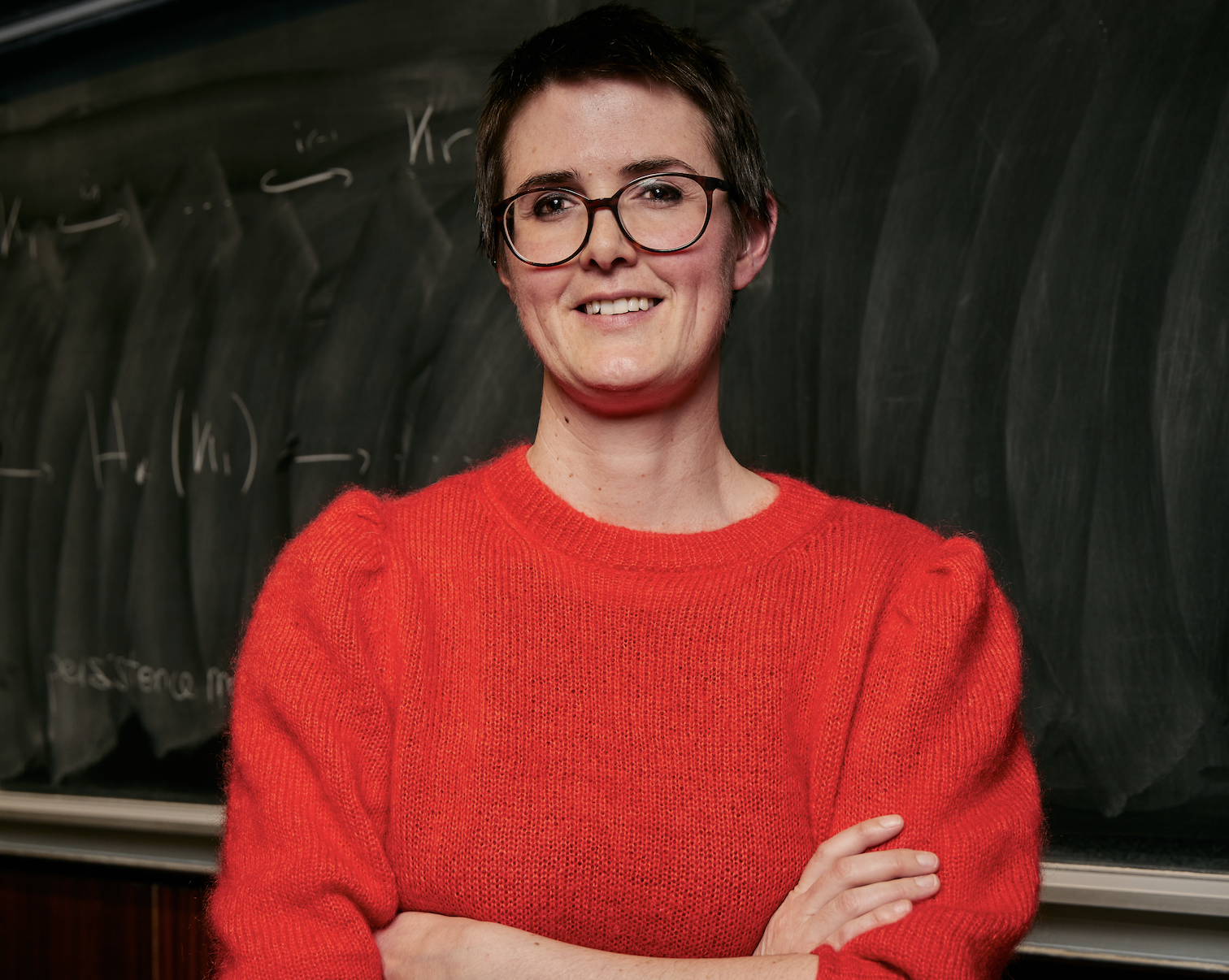
Nina Otter
Inria and Université Paris-Saclay
On the effectiveness of topology in data analysis
In the last decades methods from Topological Data Analysis have been successfully applied to a variety of data analysis problems, from predicting biomolecular properties, to discriminating breast-cancer subtypes, classifying fingerprints, or studying the morphology of leaves. At the same time, the reasons behind these successes are not yet well understood.
I believe that for Topological Data Analysis to remain relevant, we need to better understand why it is so successful. I will discuss work that attempts to address this question and outline suggestions on problems to be tackled by the community.
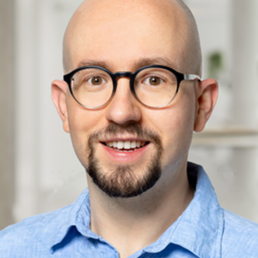
Bastian Rieck
Helmholtz - Munich
Two Households, Both Alike In Dignity: Geometry and Topology in Machine Learning
A large driver contributing to the undeniable success of deep learning models is their ability to synthesise task-specific features from data. For a long time, the predominant belief was that ‘given enough data, all features can be learned.’ However, as large language models are hitting diminishing returns in output quality while requiring an ever-increasing amount of training data and compute, new approaches are required. One promising avenue involves focusing more on the modelling aspects, developing novel inductive biases such as invariances that cannot be readily gleaned from the data. This approach is particularly useful for data sets that model real-world phenomena, as well as applications where data availability is scarce. Given their dual nature, geometry and topology provide a rich source of potential inductive biases. In this talk, I will present novel advances in harnessing multi-scale geometrical-topological characteristics of data. A special focus will be given to show how geometry and topology can improve representation learning tasks. Underscoring the generality of a hybrid geometrical-topological perspective, I will furthermore showcase applications from a diverse set of data domains, including point clouds, graphs, and higher-order combinatorial complexes.
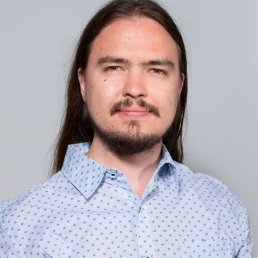
Egor Shelukhin
University of Montreal
Barcodes and iterations of Hamiltonian diffeomorphisms
We explain how to use barcodes to study Hamiltonian diffeomorphisms in symplectic topology, and how to use power operations in Floer cohomology to study their behavior under iterations, along with a few applications of these methods.
Shmuel Weinberger
University of Chicago
PH(X^Y): Some small worlds
I will discuss some results about the persistent homology of the Lipschitz constant viewed as a functional on function spaces. The small world phenomenon in sociology is the tendency of social networks to have small diameter (say linear or sublinear in log(#vertices)). We will see some similar phenomenon in (some) function spaces. While I believe that these ideas have applications, I cannot justify this belief — so people should expect a pretty pure talk.
Short Talks
Dr David Beers
Fibers of Point Cloud Persistence
How much information is lost when we apply the persistence map PH? We investigate this question by studying the subspace of point clouds with the same barcodes (from the Čech or Vietoris-Rips filtration). We establish upper and lower bounds on the dimension of this space, and find that the question of when the persistence map is identifiable has close ties to rigidity theory. For example, we show that a generic point cloud being locally identifiable under Vietoris-Rips persistence is equivalent to a certain graph being rigid on the same point cloud.
Katherine Benjamin
Multiscale topology classifies cells in subcellular spatial transcriptomics
Spatial transcriptomics technologies produce gene expression measurements at millions of locations within a tissue sample. An open problem in this area is the inference of spatial information about single cells. Here we present a multiscale machine learning method to pinpoint the locations of individual sparsely dispersed cells from subcellular spatial transcriptomics data. We integrate this approach with multiparameter persistence landscapes, a state of the art tool in topological data analysis, to identify a loop structure in infiltrating glomerular immune cells in a mouse model of lupus nephritis. Joint work with colleagues in the Mathematical Institute and Centre for Human Genetics at the University of Oxford, and the Beijing Genomics Institute.
Dr Ximena Fernandez
Let it Be(tti): applying persistent homology in the music industry
Ever wondered how music recognition apps like Shazam work or why they sometimes fail? Can algebraic topology improve current audio identification algorithms? In this talk, I will discuss recent collaborative work with Spotify, where we extract low-dimensional homological features from audio signals for efficient song identification. I will show how our method improves accuracy and reliability in matching audio content with complex distortions, outperforming Shazam.
Iolo Jones
Dr Vadim Lebovici
Local characterization of block-decomposability for multiparameter persistence modules
I will present a recent algebraic characterization of interval-decomposability of n-parameter persistence modules for a subclass of interval summands called *block modules* obtained with Jan-Paul Lerch and Steve Oudot. This structure theorem takes the form of a *local characterization*, in the sense that checking block-decomposability can be done by examining block-decomposability of restrictions of the module to elementary axis-aligned cubes. Local conditions have been proposed in the 2-parameter setting, notably for the class of block modules, which plays a prominent role in levelset persistence. Our result generalizes these 2-parameter local conditions and, to our best knowledge, it is the first interval-decomposition result in the generality of pointwise finite-dimensional modules over finite products of arbitrary totally ordered sets.
Abhinav Natarajan
Quantifying Spatial Relationships with Chromatic Delaunay Filtrations
Point cloud datasets often come with a partition of the data points, or a labelling, where one is interested in quantifying the spatial relationships between the labelled classes. In this talk I will introduce the chromatic Delaunay-Cech and chromatic Delaunay-Rips filtrations, which are novel and computationally efficient tools for quantifying such spatial relationships. I will also describe their connection to some existing approaches, including the recently developed chromatic alpha filtration [1]. [1] Sebastiano Cultrera di Montesano et al. “Chromatic Alpha Complexes”. Feb. 7, 2024. arXiv: 2212. 03128.
Otto Sumray
The Spectra of Persistence Modules
By considering an inner product between simplices, one can enhance persistence modules with geometric information. The result is known as a harmonic persistence module. However, these Euclidean representations of quivers are of wild type, even for one-parameter persistence. Therefore, seeking a decomposition into indecomposables (as with barcodes) is infeasible. In this talk I describe how the spectrum of the quiver Laplacian, introduced in previous work with Nanda and Harrington, provides an appropriate invariant of harmonic persistence modules that is able to distinguish geometric changes in topological features that ordinary persistent homology cannot see.
Tom L
The Alan Turing Institute
Some mathematical challenges in AI/ML security
I will talk about some of the mathematical challenges behind AI/ML security, including: (i) model security, i.e. structure and detection of interference; (ii) data security, i.e. data representation inside and outside the model, and the protection and provenance of data; and (iii) learning security, i.e. security challenges for the training and optimisation of models.