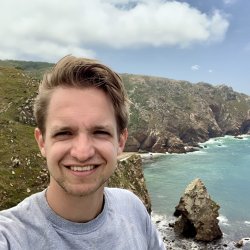
Dr Konstantin Riedl
Pronouns
He / Him
Status
Postdoctoral Research Associate
Research groups
Address
Mathematical Institute
University of Oxford
Andrew Wiles Building
Radcliffe Observatory Quarter
Woodstock Road
Oxford
OX2 6GG
University of Oxford
Andrew Wiles Building
Radcliffe Observatory Quarter
Woodstock Road
Oxford
OX2 6GG
Major / recent publications
Journal Publications
on neural PDEs:
- "Global Convergence of Adjoint-Optimized Neural PDEs" (with J. Sirignano and K. Spiliopoulos). arXiv preprint arXiv:2506.13633, 2025.
on consensus-based optimization:
- "Consensus-based optimization methods converge globally" (with M. Fornasier and T. Klock). SIAM Journal on Optimization, 2024.
- "On the global convergence of particle swarm optimization methods" (with H. Huang and J. Qiu). Applied Mathematics & Optimization, 2023.
- "Leveraging memory effects and gradient information in consensus-based optimisation: On global convergence in mean-field law". European Journal of Applied Mathematics, 2024.
- "Consensus-based optimization for saddle point problems" (with H. Huang and J. Qiu). SIAM Journal on Control and Optimization, 2023.
- "Consensus-Based Optimization with Truncated Noise". (with M. Fornasier, P. Richtárik, and L. Sun) European Journal of Applied Mathematics, 2024.
- "CB2O: Consensus-Based Bi-Level Optimization". (with N. García Trillos, S. Li, and Y. Zhu) arXiv preprint arXiv:2411.13394, 2024
- "Defending Against Diverse Attacks in Federated Learning Through Consensus-Based Bi-Level Optimization". (with N. García Trillos, A. Kumar Akash, S. Li, and Y. Zhu) Philosophical Transactions of the Royal Society A: Mathematical, Physical and Engineering Sciences, 2025.
Conference Publications
on consensus-based optimization:
- "Convergence of anisotropic consensus-based optimization in mean-field law" (with M. Fornasier and T. Klock). International Conference on the Applications of Evolutionary Computation (Part of EvoStar), 2022.
- "How Consensus-Based Optimization can be Interpreted as a Stochastic Relaxation of Gradient Descent" (with M. Fornasier, T. Klock, and C. Geldhauser). ICML Workshop on Differentiable Almost Everything: Differentiable Relaxations, Algorithms, Operators, and Simulators, 2024.
Code:
- "CBX: Python and Julia packages for consensus-based interacting particle methods". (with R. Bailo, A. Barbaro, S. N Gomes, T. Roith, C. Totzeck, U. Vaes) Journal of Open Source Software, 2024.
Theses:
- "Mathematical Foundations of Interacting Multi-Particle Systems for Optimization". Dissertation, 2024.
For a complete and up-to-date list of my publications, please see here.
Research interests
Konstantin is a Postdoctoral Research Associate in Deep Learning at the Mathematical Institute of the University of Oxford.
His research interests lie in applied mathematics with a particular focus on the design and numerical analysis of algorithms and methods used in machine learning, data analysis and signal processing. To understand their very often intricate behavior, he employs tools from PDE analysis and stochastic analysis together with numerical simulations.
Conferences
This year you can find me at the following conferences and workshops:
Physics for AI Workshop, Oxford (March 19-21, 2025)- EUROPT, Southampton (June 29-July 2, 2025)
- SIAM Annual Meeting, Montreal (July 28-August 1, 2025)
- SIAM Conference on Control and Its Applications, Montreal (July 28-August 1, 2025)
- ENUMATH, Heidelberg (September 1-5, 2025)
- Conference on Mathematics of Machine Learning, Hamburg (September 22-25, 2025)
- Workshop on Mathematics of Transformers, Hamburg (September 26, 2025, co-organizer)