Modelling the demand transfer and the halo effect in retail
- Researcher: Yu Tian
- Academic Supervisor: Renaud Lambiotte
- Industrial Supervisors: Alisdair Wallis, Sebastian Lautz
Background
The retail sector involves a huge turnover but relatively small margins, which together make the profitability very sensitive to small changes of the range of products on sale. It is then key for any retailers to acquire a quantitative understanding of the mechanisms associated with product sales. Two common ones are “demand transfer”, where removing a product leads to an increase of the sales of other product as customers consciously or unconsciously choose a substitute for this item, and “halo effect”, where the rise in sales of a product translates into increased sales of other item due to their effective dependences and associations, which might then lead to cascading effects.
There is an inherited network structure underlying the basket-level data, the product-purchase network where each transaction is connected with the products included. How products are connected through transactions and other products can then show insights in the mechanisms among them. We will start our analysis from this network.
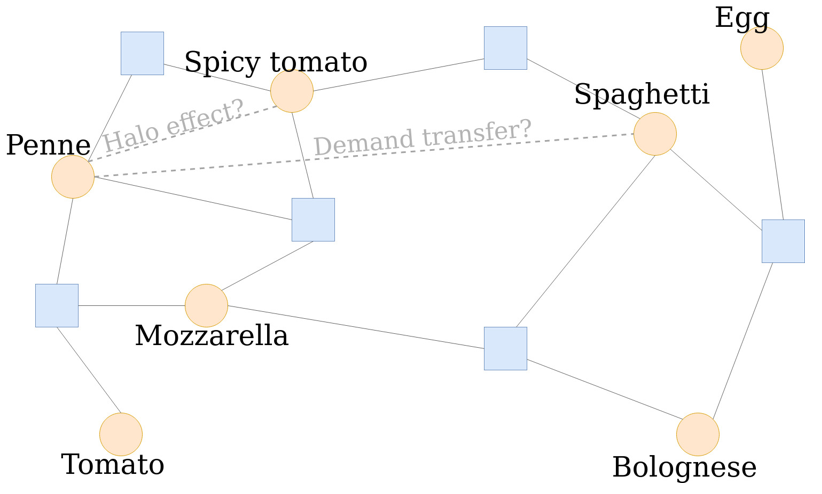
Figure 1: Example of a product-purchase network of 5 products and 6 purchases, where possible halo effect and demand transfer may exist.
The goal of this project is then to develop a data-driven network-based model on the demand transfer and the halo effect, which can be used to predict the changes in sales when manipulating the range of products, further to achieve optimal diversity of the range.
Progress
As a starting point, to quantify the halo effect, we propose structural measures for the product nodes based upon how much they are bought together. Equipped with these measures, we build a simple product network, and the demand transfer between products is quantified through the interaction with the halo effect. Hence, the problem of detecting products with strong halo effect or demand transfer is then to extract nodes with specific connectivity patterns, i.e. role extraction in networks. Specifically, these two types of roles are assortative communities, where links mostly exist within communities, and disassortative communities, where links mostly exist between communities.
Classical community detection only involves assortative communities, and can be solved efficiently. While role extraction, a more general problem, still lacks efficient solutions. To bridge this gap, and with our particular interest in disassortative communities in mind, we solve this combinatorial optimisation problem by adapting the ideas from an fairly efficient community detection method, the map equation, and modifying it to serve our purpose. We have shown the effectiveness of our method through analysing its resolution limit, and the robustness through studying the eigenspace of the adjacency matrix. Experimentally, our method also successfully extends to the disassortative regime, and outperforms others in terms of a larger detecting region (around 50% more in a planted two-block stochastic block model).
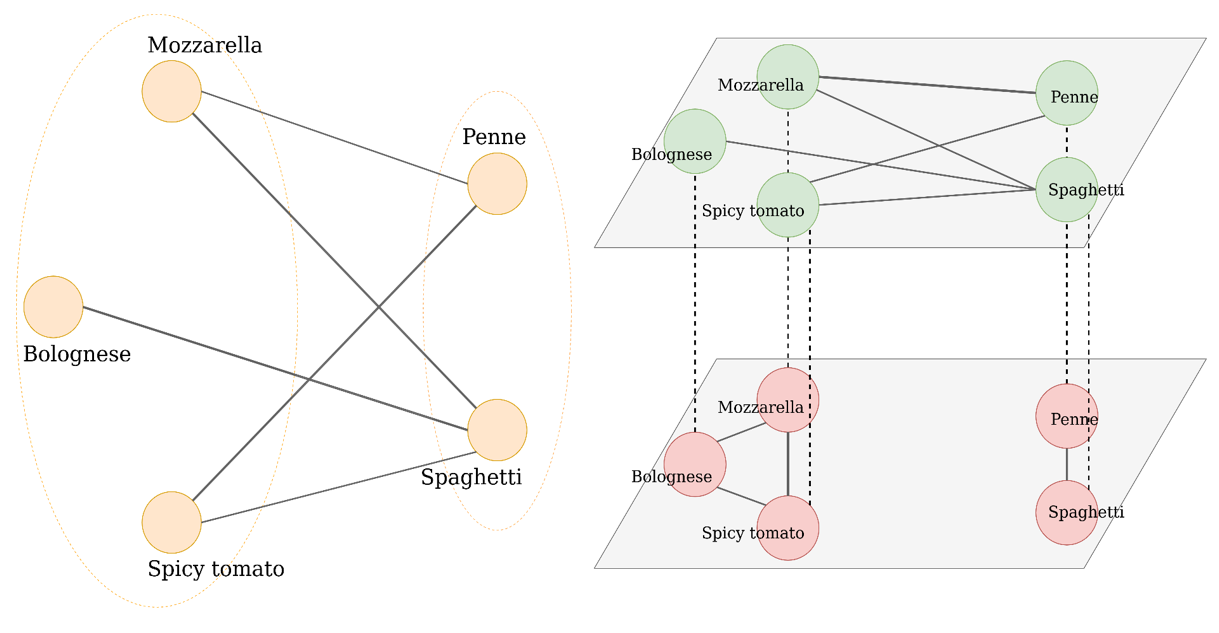
Figure 2: Example of a constructed (left) simple network where the halo effect is shown through direct neighbourhoods and products with high demand transfer are shown in the dashed circles, and (right) two-layer network where the top layer shows the halo effect and the bottom layer shows the demand transfer.
As a next step, with more assumptions on the interaction between the halo effect and the demand transfer, we aim to use more than the direct neighbourhood of product nodes, and propose measures for both mechanisms. Both measures are solved simultaneously through an iterative method. To leave more freedom for the interacting effect between the halo effect and the demand transfer, we model them as a two-layer network, as shown in Fig. 2, where groups of products with high halo effect or demand transfer can then be detected.
Future Work
Our future work involves extending the role extraction method to multilayer networks, and proposing methods for extracting roles in bipartite networks directly. Since noise plays a significant role in real data analysis, we also plan to systematically analyse the noise effect at the node, mesoscale and global level, especially on our measures.