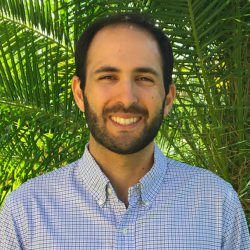
Prof. Justin Sirignano
University of Oxford
Andrew Wiles Building
Radcliffe Observatory Quarter
Woodstock Road
Oxford
OX2 6GG
Please see my CV for a complete list of publications. Some example publications are listed below.
- "Mean Field Analysis of Neural Networks: A Law of Large Numbers" (with K. Spiliopoulos). SIAM Journal on Applied Mathematics, 2020.
- "Mean Field Analysis of Deep Neural Networks" (with K. Spiliopoulos). Mathematics of Operations Research, 2021.
- "Universal features of price formation in financial markets: perspectives from Deep Learning" (with Rama Cont). Quantitative Finance, 2019.
- "Mean Field Analysis of Neural Networks: A Central Limit Theorem" (with K. Spiliopoulos). Stochastic Processes and their Applications, 2019.
- "Continuous-time stochastic gradient descent for optimizing over the stationary distribution of stochastic differential equations" (with Ziheng Wang). Mathematical Finance, 2023.
- "DPM: A deep learning PDE augmentation method with application to large-eddy simulation" (with J. F. MacArt and J. B. Freund). Journal of Computational Physics, 2020.
- "PDE-constrained Models with Neural Network Terms: Optimization and Global Convergence" (with J. F. MacArt and K. Spiliopoulos). Journal of Computational Physics, 2023.
- "Neural Q-learning for solving Elliptic PDEs" (with S. Cohen and D. Jiang). Journal of Machine Learning Research, 2023.
- "Deep Learning Closure Models for Large-Eddy Simulation of Flows around Bluff Bodies" (with J. MacArt). Journal of Fluid Mechanics, 2023.
- "Kernel Limit of Recurrent Neural Networks Trained on Ergodic Data Sequences" (with S. Lam and K Spiliopoulos). arXiv:2308.14555, 2023. Minor Revision at Annals of Applied Probability.
- "Online Optimisation of Machine Learning Collision Models to Accelerate Direct Molecular Simulation of Rarefied Gas Flows" (with N. Ball and J. MacArt). arXiv:2411.13423, 2024.
- "Convergence Analysis of Real-time Recurrent Learning (RTRL) for a class of Recurrent Neural Networks" (with S. Lam and K. Spiliopoulos). arXiv:2501.08040, 2025.
Courses: Deep Learning, Machine Learning, Partial Differential Equations, Stochastic Models, Financial Mathematics
Course director of the Oxford Masters program in Mathematical & Computational Finance (55 students per year, recently ranked #1 Mathematical Finance programme in the UK)
Career Placement of PhD Students: Lei Fan (J.P. Morgan Systematic Trading), Xiaobo Dong (J.P. Morgan Machine Learning), Deqing Jiang (Bank of America Fixed Income)
Ranked Excellent in Teaching for a graduate course on deep learning with two hundred students.
Justin is a Professor of Mathematics at the University of Oxford whose research focuses on Applied Mathematics, Machine Learning, and Financial Mathematics. His research has developed new mathematical theory and numerical methods in finance, deep learning, and scientific machine learning.
He has contributed to the mean-field analysis of deep learning models, including multi-layer neural networks, reinforcement learning, and recurrent neural networks. Another area of interest has been the development and rigorous mathematical analysis of machine learning methods for stochastic differential equations (SDEs) and partial differential equations (PDEs).
In parallel, Justin's research group and collaborators have worked on several application areas. In mathematical finance, they have developed deep learning models for large financial datasets such as: high-frequency data from limit order books, loans, and options. He is also developing deep learning methods for constructing PDE models from data, which has a variety of applications in science, engineering, and finance. This includes recent work in the area of "scientific machine learning" on developing deep learning closures for reduced-order PDE models (e.g., RANS and LES) of computationally-challenging physics (e.g., turbulence and hypersonics).
Justin received his PhD from Stanford University and holds a Bachelors degree from Princeton University. He was a Chapman Fellow at the Department of Mathematics at Imperial College. He was awarded the 2014 SIAM Financial Mathematics and Engineering Conference Paper Prize. His research has been funded by agencies such as EPSRC, ONR, and DoE.
E-Mail: @email
See my CV for a complete list of publications, grants, and presentations.
Grants:
Office of Naval Research (ONR): $1 million grant in collaboration with Jonathan MacArt (Notre Dame) and Marco Panesi (UIUC)
NSF-EPSRC: $750,000 grant in collaboration with Jonathan MacArt (Notre Dame)
DMS-EPSRC: $847,000 grant in collaboration with Konstantinos Spiliopoulos (Boston University)
Other Industrial and Government funding: 1 postdoctoral researcher (2 years), 3 PhD students (4 years each)
Co-I for EPSRC Hub for Mathematical and Computational Foundations of AI (2024-)
Co-PI on $16.5 million DoE Center for Exascale-enabled Scramjet Design at UIUC (2020-2021).
Recent computational grants:
8 million GPU hours from DoE's Innovative and Novel Computational Impact on Theory and Experiment (INCITE) program (2025)
1.5 million GPU hours from DoE's SummitPlus program (2024)
160,000 GPU hours from EPSRC (2024)
3 million GPU hours from the DoE's INCITE program (2023)