Sampling Methods for Large Networks and Application to International Research Dynamics
- Researcher: John Fitzgerald
- Academic Supervisors: Peter Grindrod, Neave O’Clery
- Industrial Supervisor: Rachel Herbert
Background
A consensus has recently emerged to support the role of capability and knowledge accumulation in economic development. Specifically, countries move into new economic activities as their workforce learns new skills and upgrades its capability base. This process of capability accumulation can occur through a variety of channels, including on-the-job learning, inward migration, and (most importantly here) research collaboration.
We are specifically interested in this third channel, namely the process by which academics tap into new strands of research, begin to collaborate with influential academics located in developed contexts, and potentially relocate to centres of academic excellence (temporarily or otherwise). This migratory training path is well-established amongst developing countries. Our aim is to develop a model to describe the knowledge accumulation process by which these academics manage to create new centres of research excellence in the developing world, through forming and analysing temporal networks from publication data. This is particularly important as the geography of academic production is highly uneven globally, as displayed in Figure 1 below.
Figure 1: A world map where countries are coloured by the natural logarithm of the number of publications they produced in 2018.
This research will form one part of the evidence base generated by Elsevier’s new International Centre for the Study of Research, which aims at understanding the dynamics of knowledge accumulation worldwide. We hope to contribute to the development of Elsevier’s global strategy in assisting the emergence of new academic markets.
Progress
We have so far focused on applying contemporary network science techniques to the graphs formed by international coauthorship relations between states over time. We have applied a state-of-the-art community detection algorithm to the networks formed from aggregating these graphs into five-year slices to produce Figure 2 below.
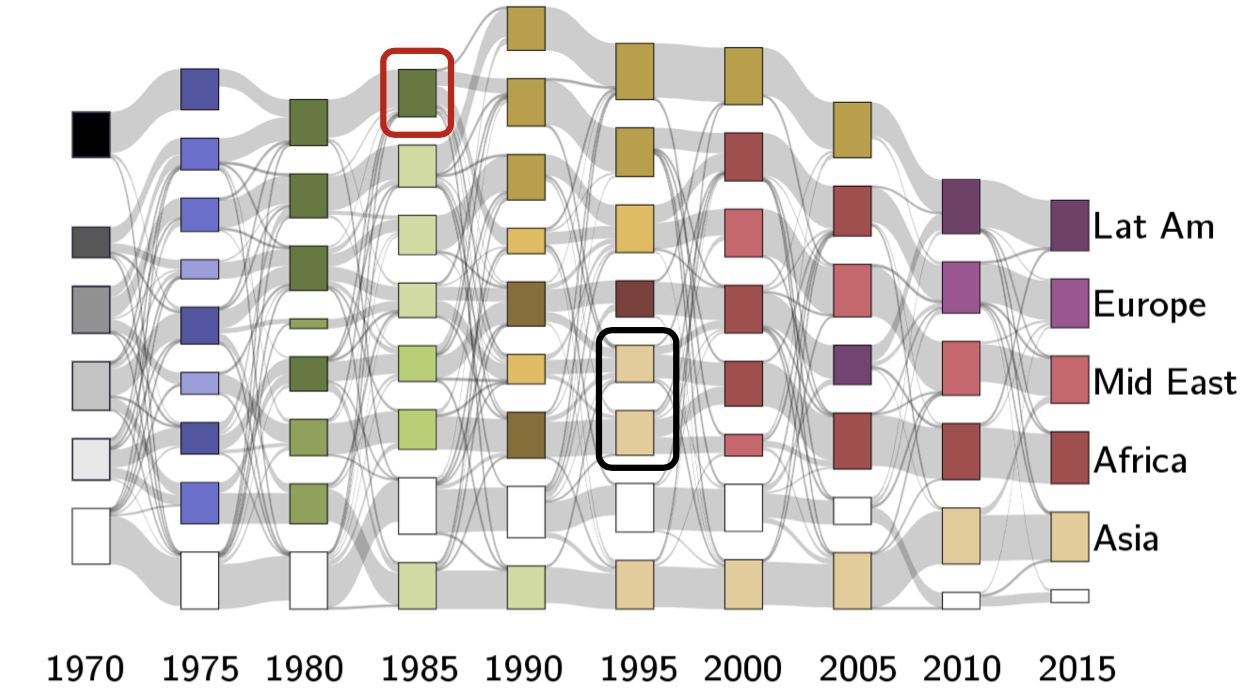
Figure 2: Community detection as applied to international coauthorship relations between states over time, where blocks are communities (coloured for distinction) and temporal flow between communities is sized according to Jaccard index (the number of elements common to both sets divided by the total number of unique elements between them).
Inspecting Figure 2 we may observe several historical events, such as the breakup of the Soviet Union (highlighted in red) and the decreasing significance of post-colonial countries and their previous imperial cores (highlighted in black). This preliminary study emphasises the interrelated nature of geopolitics and academic collaboration, and provokes much further study for policy recommendations, such as the impact on national economic indicators such as Gross Domestic Product (GDP).
Future Work
A crucial next step is to disaggregate the network to the institutional and even individual level, to better understand how regional inequalities and specialties affect knowledge production, and determine areas and institutions that are particularly successful given their circumstances. To better understand structural changes in the network, and possible causes for these changes, we will fit probabilistic models and apply Bayesian criterions for model evaluation. The large size of the global academic network and complexities of the models applied require the development of sampling based approaches to improve computation time, and allow us to incorporate prior uncertainty into forecasts of network evolution in a pragmatic fashion.