Community Detection in Customer-Store Networks
Researcher: Rodrigo Leal Cervantes
Academic Supervisors: Renaud Lambiotte and Sam Howison
Industrial Supervisors: Rosie Prior and Jason O'Sullivan
Background
Most customers have a preferred retailer (e.g. Tesco, Sainsbury’s or Waitrose) which they visit across multiple physical stores and possibly also online. The brick-and-mortar stores that any one customer visits often have different formats (convenience, high-street, hypermarket, etc.) with their own size, characteristics (such as parking space) and product offering. Each store fulfils different shopping needs and the result is that customers shop for groceries across a network of stores.
Through our research partnership with Dunnhumby — a global Customer Data Science company that works with leading retailers to develop loyalty schemes and deliver personalised customer experiences — we aim to study and model the customer-store network belonging to one of the largest retailers in the UK.
We are particularly interested in studying the network through the lens of community detection — a set of tools that allow us to partition the network into groups and find its building blocks. Our goal is to develop useful methods for extracting groups of customers and stores that play similar roles, taking into account that the networks are embedded in physical space and that proximity is a crucial factor when customers decide where to shop.

Figure 1: Validation using a synthetic network: the nodes are placed uniformly inside a square and divided into two planted communities; we generate the links by connecting preferentially nodes within the same group but also pairs of nodes that are located in close proximity. In our experiments, we build the spatial backbone and we keep only those edges that have a significantly large or small weight which then reveals the block structure more clearly. By fitting an SBM to these new networks, we perfectly recover the planted communities.
Progress
One of the main tools that we use is a statistical model for networks called the stochastic block-model (SBM). Previously, we worked on a theoretical question about clarifying the relationship between a type of SBM and a popular measure used to find communities called modularity. We adapted a method for modularity maximisation, the Louvain algorithm, and used it to maximise a marginal-likelihood function for the SBM (including a prior distribution that limits the number of communities found).
Having built our understanding on the inner workings of the SBM, we focused on the problem of adapting it to study a network that is embedded in physical space. This is challenging because inside the standard SBM there is no way to account for coordinates and there is no notion of distance or proximity between pairs of nodes.
Our solution is to first analyse the spatial network using population-level human mobility models that tell us what is the level of interactions that would be expected between pairs of locations, taking into account the distance between them. We use these predictions to determine which links are statistically significant, either because they are too large or two small, and we construct what we call the spatial backbone of the original network. We can then fit an SBM to the data to find the meso-scale organisation of the network.
To gain confidence that our method works (under the right circumstances) we have done extensive validation experiments. We use a family of synthetic networks where nodes have been divided into two groups and we define parameters that control the number or intra- and inter-group links, and where the probability of linking nodes increases if there are located close to each other. Our synthetic networks thus show a mixture of spatial and non-spatial effects which can be tuned. When we build the spatial backbone of the synthetic networks, our method discounts the spatial effects and it makes the discovery of the planted communities easier, as attested in Figure 1.
When we use our method to study the retail data, we find a complex pattern of interactions between the stores. Some of the groups seem to be correlated with the format of the stores (for example, in Figure 2, we find many groups that are composed mostly of Petrol stations all across the UK), and others seem to relate to travel hubs like airports and train stations. We see a core-periphery structure around London and the metropolitan area around Manchester, but also also in coastal areas like Norfolk or Cornwall.
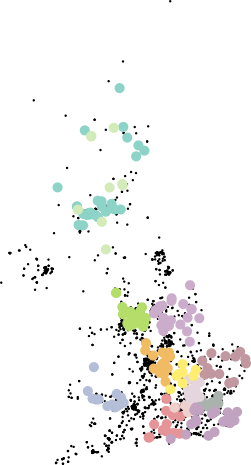
Figure 2: Subset of the communities found: we highlight groups of stores that are found by fitting an SBM to the spatial backbone. The highlighted groups are primarily composed of petrol stations, showing that these stores play a unique role inside the mobility network.
Future Work
We plan to build upon our work and study the mobility of individual customers, inferred from the stores they visited up to a given point. This complements the study we have done so far with human mobility models that are aggregated for a large population, since we will now focus on individual mobility models. One of the goals of this analysis will be to cluster the customers according to their mobility profiles, and another one will be to characterise any patterns that can be exploited for predicting where a customer could shop next.