Early detection of exacerbation events from telemonitoring data using path signatures.
- Researcher: James Morrill
- Academic Supervisor: Terry Lyons, Sam Howison
- Industrial Supervisor: Sumanth Swaminathan
Iterex Therapeutics
Background
Conditions such as congestive heart failure (CHF), asthma, and chronic obstructive pulmonary disease (COPD) are a constant burden to patients' daily lives. Patients are subject to:
- Acute exacerbations – sudden flare-ups that require increased medical attention and treatment; leading to
- Long-term decline in patient physiological function, recurrent distress/anxiety attacks, and significant economic burden.
Existing tools for detecting and managing escalation of chronic illness at home often using a rule based ‘action plan checklist’:
- These do not adequately capture the complexity and diversity of such health episodes and
- can result in a delay in receiving treatment, leading to more adverse health outcomes.

Iterex Therapeutics specialises in at-home digital therapies – software applications that utilise machine learning to improve diagnosis of, and response to exacerbation events. These applications have been deployed in patient clinical trials where patient data is collected on a daily or weekly basis.
The goals of this project are as follows:
- Develop an early warning system for signs of exacerbation.
- Interpret the models to develop an understanding of the processes and interactions that take place during an exacerbation.
- Create a set of tools for the analysis of anomaly detection problems in the small data regime.
Progress
To develop a better understanding of the methodologies and techniques to be used in the main project, we considered a problem in stress detection. We used the publicly available Wearable Stress and effect Detection dataset (WESAD) where patients were induced to experience stressed and non-stressed states. Breathing, electrodermal activity and electrocardiogram measurements were collected at a sampling rate of 700Hz. The original paper describing the study method also provided a baseline model for predicting the stressed vs non-stressed states. Accuracy achieved in discriminating between the two states was 93.12%.
The key tool used to perform the analysis of these data streams will be the path signature. A signature is a mathematical transformation which can be applied to a data stream to convert a path into a manageable set of summary statistics. In our case, the path is made up of the Resp, EDA and ECG signals as they change over time. These summary statistics can then be fed into our learning algorithms as features. The statistics surmised by the signature are very good at describing the shapes of the paths in fine detail. We expect this to be important in our problem due to the presence of breathing data. Additionally, the volume of breath inhaled and exhaled is likely to have key differences in stressed vs non-stressed scenarios.
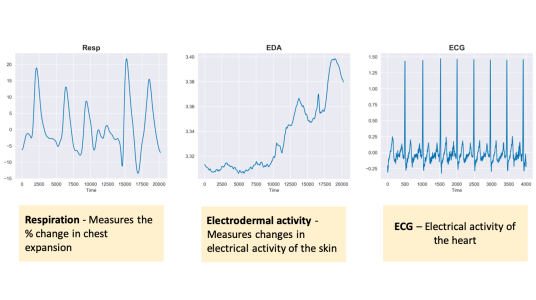
Through a combination of domain expertise and knowledge about how features are extracted from the path signature, the paths were preprocessed into forms from which useful features related to stress could be easily extracted. After employing these path signatures, we then developed and implemented an algorithm which results in significant improvements over the current state-of-the-art stress detection algorithms. Our algorithm can accurately predict 98.05% of stressed/non-stressed states whereas current state-of-the-art stress detection algorithms can only predict 93.12% correctly.
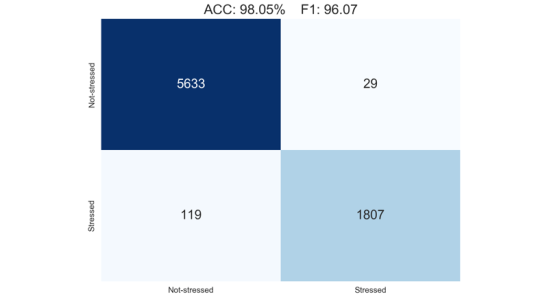
Future Work
We have ongoing work developing algorithms for the early detection of sepsis to improve sepsis outcomes. The data is sourced from ICU patients in three separate hospital systems and contains information for 8 regularly sampled vital signs, 25 infrequently sampled laboratory measured values, and patient demographics. Around 9% of patients end up developing sepsis at a median time of 35 hours. We have been uncovering interesting feature relationships and sepsis development information that we are working on turning into predictive power, whilst keeping the methodologies quite general so that they can be easily applied to other similar problems such as our main focus on exacerbation detection.