Scientific Growth: from Research to Field
Researcher: Ambrose Yim
Academic Supervisors: Prof Peter Grindrod, Dr Andrew Mellor
Industrial Supervisor: Kate Hibbert
Elsevier
Background
Elsevier are traditionally a publishing company, publishing more than 430,000 articles annually in 2,500 journals. More recently Elsevier have shifted towards a scientific analytics model, aiming to the be industry leader in academic data and the science of science. In the course of developing products for researchers, Elsevier need meaningful and useful descriptors of a researcher’s scientific journey and methods of contextualising a researcher’s output in the wider academic field.

One important dynamic in research is the closure of of gaps or holes in bodies of knowledge. Though we have considerable data on publications and author profiles, information such as text, citation and co-authorship is heterogeneous, opaque and difficult to parse algorithmically. Recent advances in machine learning on natural language processing have allowed us to overcome some challenges. Topic modelling methods allow us to represent a collection of texts as a point cloud in a low dimensional Euclidean space, making them amenable to other data science methods. The leading method for analysing holes in point clouds is persistent homology, which detects the birth and death holes in the point cloud as the point cloud is coarse-grained on larger and larger length scales. Persistent homology allows us to mathematically quantify holes in knowledge. By overlaying information from persistent homology onto other meta data, we may discover correlations between other meta data and the closure of gaps in research.
Progress
We have so far focused on extracting the dynamics of research trends from a body of papers. The impact is two fold. On a macroscopic level, we can observe the interaction of different schools of thought within a body of research and how innovation is borne out of such interactions. On the level of a researcher, we can contextualise a researcher’s output by analysing their input in various trends. We have applied a state-of-the-art algorithm for abstracting time-evolving structure in point clouds, known as the Mapper algorithm, to papers from the Journal of Machine Learning Research to generate a time-directed graph from an embedding of papers, where the time-directed edges represent a continual publication of papers in a similar topic area over time, i.e. a research trend. We then used extended persistent homology to extract the homological critical points of the Mapper graph, i.e. the forks, mergers and recombination of research trends over time. This procedure allows us to filter papers that play an important role in these critical interactions between research trends for further analysis.
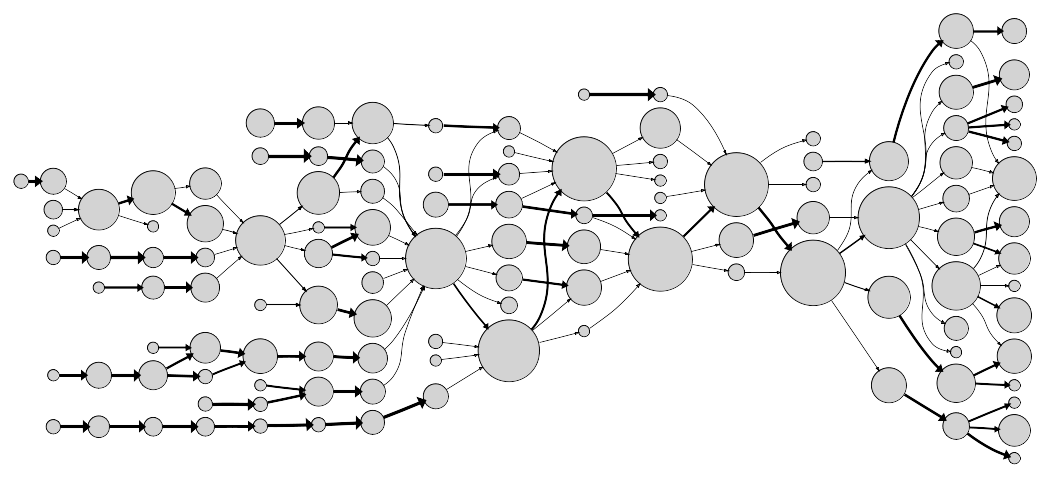
Future work
We foresee that the mapper algorithm can be applied to analysing the research output of academic institutes to identify interdisciplinary interactions between faculties and departments. To extend our methodology, we will be applying the persistence vineyards algorithm to evolving point clouds, which allows us to track the changes in the scale of holes in the point cloud as time evolves, giving us a richer description of the closure of gaps and holes in research.